
Table: for males, the odds of being in the honors class are (17/91)/(74/91) =ġ7/74 =. Of a female being in the honors class? We can manually calculate these odds from the In our dataset, what are the odds of a male being in the honors class and what are the odds Look at the crosstab of the variable hon with female. Logit(p) = β 0 + β 1* female Logistic regression Number of obs = 200 Writing it in an equation, the model describes the Now let’s go one step further by adding a binary predictor variable,įemale, to the model. Logistic regression with a single dichotomous predictor variables Predictor variables is the estimated log odds of being in honors class for the whole populationĬan also transform the log of the odds back to a probability: p = exp(-1.12546)/(1+exp(-1.12546)) = In other words, the intercept from the model with no The overall probability of being in honors class ( hon = 1). Logit(p)= β 0 Logistic regression Number of obs = 200 Let’s start with the simplest logistic regression, a model without any

Logistic regression with no predictor variables The output on this page was created using Stata with someĮditing. Purposely ignore all the significance tests and focus on the meaning of the Set has 200 observations and the outcome variable used will be hon, indicating if a student is inĪn honors class or not. Use a sample dataset,, for the purpose of illustration. We are now ready for a few examples of logistic regressions. More formally, let $Y$ be the binary outcome variable indicating failure/success with $\.$$ It models the logit-transformed probability as a linear relationship with the predictor variables. The other common choice is the probit transformation, which will not be covered here.Ī logistic regression model allows us to establish a relationship between a binary outcome variable and a group of predictor This transformation is called logit transformation. Another reason is that among all of the infinitely many choices of transformation, the log of odds is one of the easiest to understand and interpret. It maps probability ranging between 0 and 1 to log odds ranging from negative This transformation is an attempt to get around the restricted range problem. Why do we take all the trouble doing the transformation from probability to log odds? One reason is that it is usuallyĭifficult to model a variable which has restricted range, such as probability. We have also shown the plot of log odds against odds. The table below shows the relationship among the probability, odds and log of odds. That is to say, the greater the odds, the greater the log of odds and vice versa. Again this is a monotonic transformation. The transformation from odds to log of odds is the log transformation (In statistics, in general, when we use log almost always it means natural logarithm). Below is a table of the transformation from probability to odds and we have also plotted for the range of p less than or equal to. The transformation from probability to odds is a monotonic transformation, meaning the odds increase as the probability increases or vice versa.

5, i.e., 50-50 percent chance, then the odds of success is 1 to 1. That is to say that the odds of success are 4 to 1. The odds of success are defined as the ratio of the probability of success over the probability of failure. Let’s say that the probability of success of some event is. From probability to odds to log of oddsĮverything starts with the concept of probability.
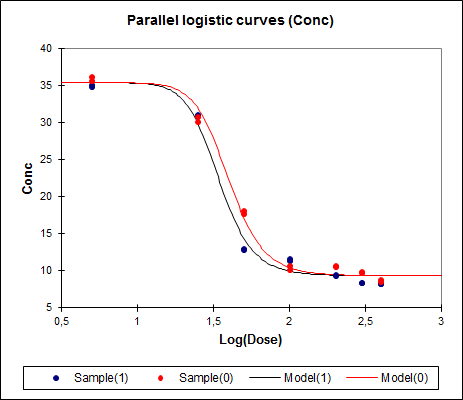
In this page, we will walk through the concept of odds ratio and try to interpret the logistic regression results using the concept of odds ratio in a couple of examples. When a binary outcome variable is modeled using logistic regression, it is assumed that the logit transformation of the outcome variable has a linear relationship with the predictor variables.
